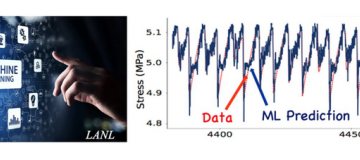
Machine Learning, Artificial Intelligence and earthquake forecasting
Speaker: Prof. Chris Marone (Università di Roma La Sapienza, Dipartimento di Scienze della Terra) - 1st Dicembre - 4,30 pm | Aula Arduino
01.12.2022
This talk will summarize how machine learning (ML) is being used with both active and passive lab seismic data to predict lab earthquakes. These works show: 1) that ML can predict the timing and magnitude of labquakes using acoustic emissions (AE) that originate in the lab fault zone and 2) that in addition to these passive measurements of lab AE, active source measurements of changes in fault zone elastic properties during the lab seismic cycle can be used to predict lab earthquakes. Our data show that labquakes are preceded by a cascade of AE events and systematic changes in elastic wave speed and transmitted amplitude that foretell catastrophic failure. The methods include traditional ML techniques based on regression, deep learning (DL) prediction of failure times and event magnitudes, and also DL-based methods to autoregressively forecast labquakes and fault zone shear stress. Such works document the evolution of frequency magnitude statistics during the lab seismic cycle, which provides an opportunity to use ML to interrogate the physics of impending failure. The data on labquake precursors provides a sensible connection between the ML-based predictions, based on AE, and the physics of failure. In the lab, AE events represent a form of foreshock and, not surprisingly, the rate of foreshock activity correlates with fault slip rate and its acceleration toward failure. A central goal of this work is to learn from lab earthquake prediction to improve forecasts of earthquake precursors and tectonic faulting. Basic questions remain regarding how slow ruptures can propagate quasidynamically, at speeds far below the Rayleigh wave speed, and how tectonic faults can host both slow slip and dynamic earthquake rupture.