The role of artificial intelligence in the forecasting of future landslide evolution
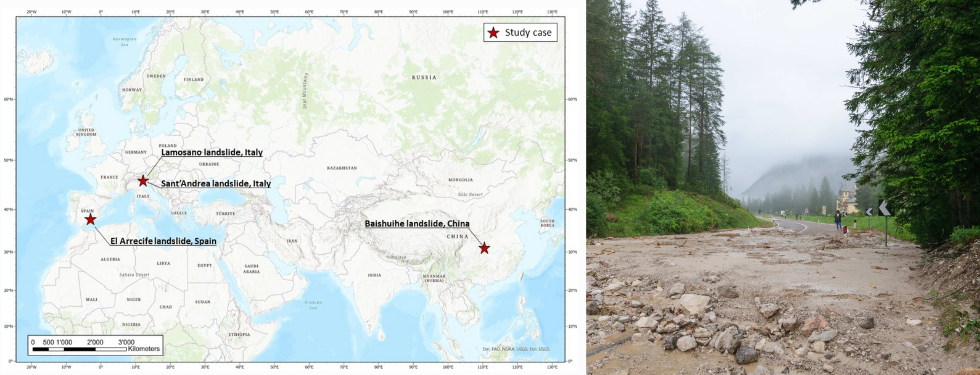
Landslides cause a tremendous number of human fatalities globally and significant economic losses every year.
Predicting the evolution of landslides is essential for risk assessment and the design of reliable early warning systems having the capability to include the impact of external triggers such as rainfall, reservoir and groundwater fluctuations, earthquakes, and anthropogenic activities. However, it can be difficult to predict how landslides will behave in the future because their progression typically does not adhere to a linear pattern.
Recent breakthroughs in landslide displacement forecasting have demonstrated that artificial intelligence (AI) and, in particular, deep learning techniques can be a turning point in designing effective early warning systems.
A study recently published in the journal Landslides and led by a team of researchers from the Department of Geosciences of the University of Padua in collaboration with colleagues from universities and research centers in Spain and Austria, tested the effectiveness of seven deep learning techniques for predicting landslide movements.
The case studies were four landslides that differ in terms of geographical location, influencing factors, geological settings, time step dimensions, and measurement sensors: the Sant'Andrea and Lamosano landslides in the Dolomites, (Italy), the Baishuihe landslide in the Hubei province, (Central China), and the El Arrecife landslide located in the Granada province (Southern Spain).
“Artificial intelligence and specifically machine learning techniques have been employed to forecast landslide displacement. But when it comes to neural networks, the analysis has never been comprehensive of different phenomena in different models altogether. In this research we decided to employ seven state of the art neural networks and four different landslides”, says Lorenzo Nava, PhD student of the Department of Geosciences of the University of Padova and main author of the study.
“Two cases are rainfall triggered and the other two cases are also triggered by the changes in the water level reservoir”, Lorenzo Nava adds.
In summary, this study has shown that deep learning (DL) can be successfully integrated to landslide early warning systems (EWS). “We found that three models demonstrated the ability to produce reliable predictions in each of the four scenarios”, Lorenzo Nava concludes.
The repository that contains all the codes and the sample data to train and optimize these seven deep learning models is available on Lorenzo Nava’s GitHub profile
Doi: https://doi.org/10.1007/s10346-023-02104-9
Title: Landslide displacement forecasting using deep learning and monitoring data across selected sites
Authors: Lorenzo Nava, Edoardo Carraro, Cristina Reyes-Carmona, Silvia Puliero, Kushanav Bhuyan, Ascanio Rosi, Oriol Monserrat, Mario Floris, Sansar Raj Meena, Jorge Pedro Galve and Filippo Catani